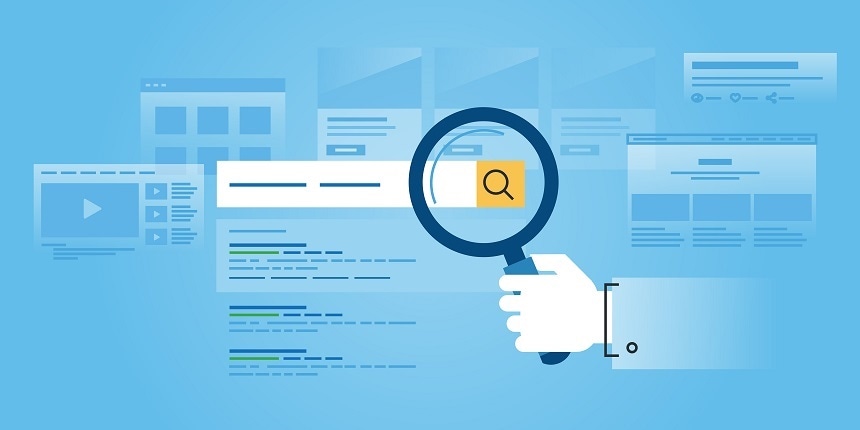
Image credit: PureSolution/Shutterstock
Search intent isn’t an advanced SEO (Search Engine Optimisation) concept. For years, digital marketers have understood that search engines – predominantly Google – use algorithmic machine learning to rank search engine results pages (SERPs) in order of relevance. This is determined by several key parameters, including the perceived intent behind a given search query. Understanding what these are, and how to cater to them, can be fundamental to success in your marketing science and SEO efforts.
In 2015, Google identified just four categories of intent that could underlie a search query at every stage in the digital consumer journey: I want to know, I want to do, I want to go, or I want to buy.
An Overview of Traditional Intent Factors
The vernacular varies, but these generally outline the four so-called micro-moments that Google identified as “game changers for both consumers and brands”. The idea of micro-moments was that beneath every query typed into Google is a primary intention, people either want the answer to a question; something to do; a site to visit; or a product to purchase. This information was a silver bullet for digital marketers. Not only could we optimize content for specific keywords, we could tailor that content for specific micro-moments to maximize its chances of success (engagement, lead-generation, ranking, etc.).
Although punchy and easy to convey in laymen’s terms, these four micro-moments really boiled down to three forms of content when it came to practical SEO. These were defined in a peer-reviewed paper by Andrei Broder as early as 2002:
- Navigational: A searcher intends to find a site.
- Informational: A searcher wants information or an answer to a question.
- Transactional: A searcher wants to purchase something; a product or service.
However, these categorizations rapidly run into problems. For one, they are far too broad and fail to prescribe for nuanced searches. A practical example might be a search for marketing science experts: is the user attempting to navigate to a specific site, or make a transaction for services? The SERPs are subsequently mixed, forming an overlap.
So, the traditional model of search intent optimization is notably flawed. Yet it still works as a decent hypothetical model to explain the content optimization process; something like a skeletal structure. New models have been proposed to help put some muscle on the bones.
AI & Natural Language: A New Landscape of Search Intent
Google crawls webpages to determine their relevance to a search term, which defines whereabouts those pages fall in the SERPs. This crawling activity is conducted by the overall Google algorithm. The algorithm was a nameless entity until 2013 when it was christened Hummingbird. It is a sum of many moving parts that continuously crawls and ranks pages based on hundreds of distinct ranking signals, from words on the page to linking structures. With the onset of artificial intelligence (AI) and natural language processing, Google introduced the machine-learning system RankBrain. The exact purpose and function of RankBrain remain unclear, but it provides fundamental information in processing SERPs. As it is often discussed in tandem with AI, experts have guessed that the new system is part of how Google SERPs have become so intuitive.
To coin a proposed definition from Search Engine Land:
RankBrain is mainly used as a way to interpret searches that people submit to find pages that might not have the exact words that were searched for.
In other words, RankBrain helps Google interpret what users want to find based on non-traditional factors (keywords, etc.). As of 2016, Google was using RankBrain for every single search processed and was fundamental in ranking many of them.
Cutting through the industry jargon and hyperbole; what does this mean for content optimization?
The improvements to query refinement (offered by RankBrain) and the improved semantic intelligence of Google, means that the SERPs are now more intelligent than the traditional micro-moment model. One model suggests that there are as many as nine different indicators of intention; breaking down navigational, informational, and transactional queries into format-related SERPs.
For example: There is a subtle difference between research-based queries and questions. Organic results for “navigational” search terms may be localised based on the user’s IP address. Two searches for the same keyword with slightly different semantic variations may yield SERPs with totally different visual information; images versus videos.
Here is a quick comparison from our industry, using the keyword ‘atomic force microscope’. We can search the keyword on its own, or we can add modifiers, like ‘best’. The difference is a totally different SERP:
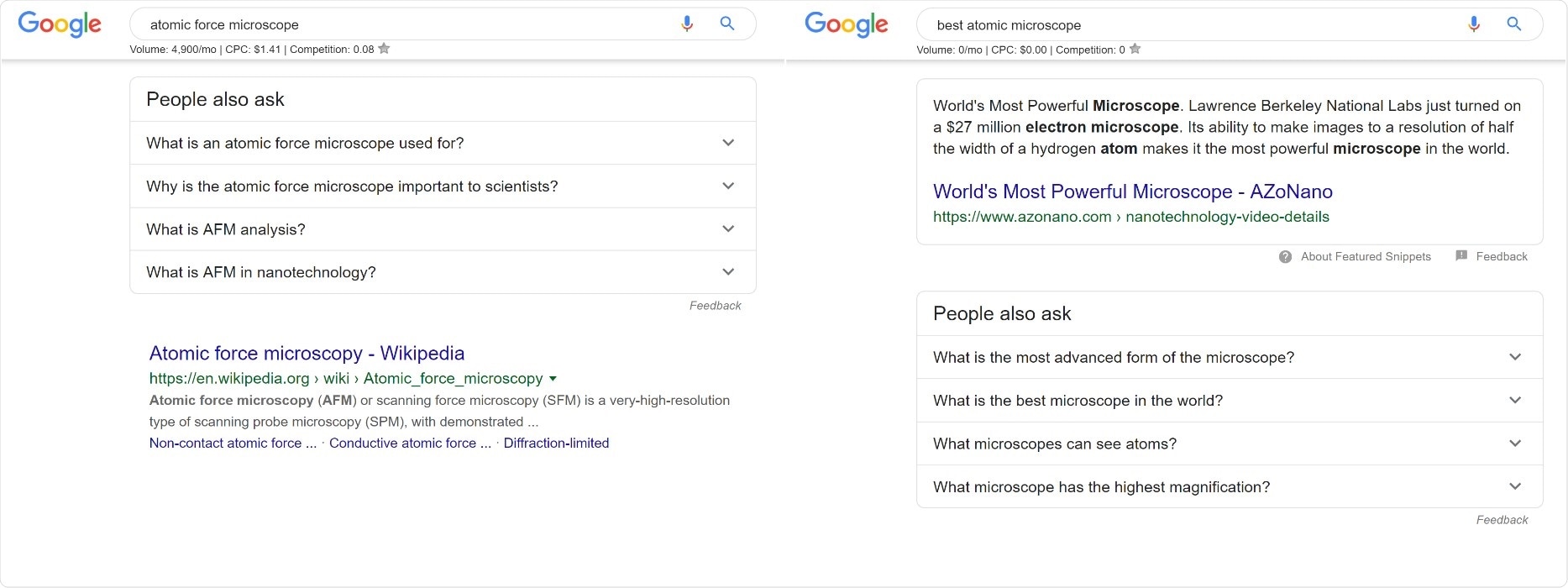
Position one changes dramatically based on the modifier 'best', a signifier of commercial intent.
It’s important to remember that digital marketers are not looking to classify, or even understand, the intentions of users. We are aiming to understand the type of content that Google ranks highly based on the search engine’s own interpretation of intent. That way we can deliver content that is tailored at an increasingly granular level, beyond those conventional micro-moments, practically guaranteeing improved rankings and user engagement.
Sources
https://www.thinkwithgoogle.com/marketing-resources/micro-moments/how-micromoments-are-changing-rules/
http://www.cis.upenn.edu/~nenkova/Courses/cis430/p3-broder.pdf
https://www.contentharmony.com/blog/classifying-search-intent/
https://searchengineland.com/faq-all-about-the-new-google-rankbrain-algorithm-234440